Reflections On FIAM Conference Discussions
Apurv Jain, CEO MacroXStudio Inc. and Bill Kelly CEO CAIA Association
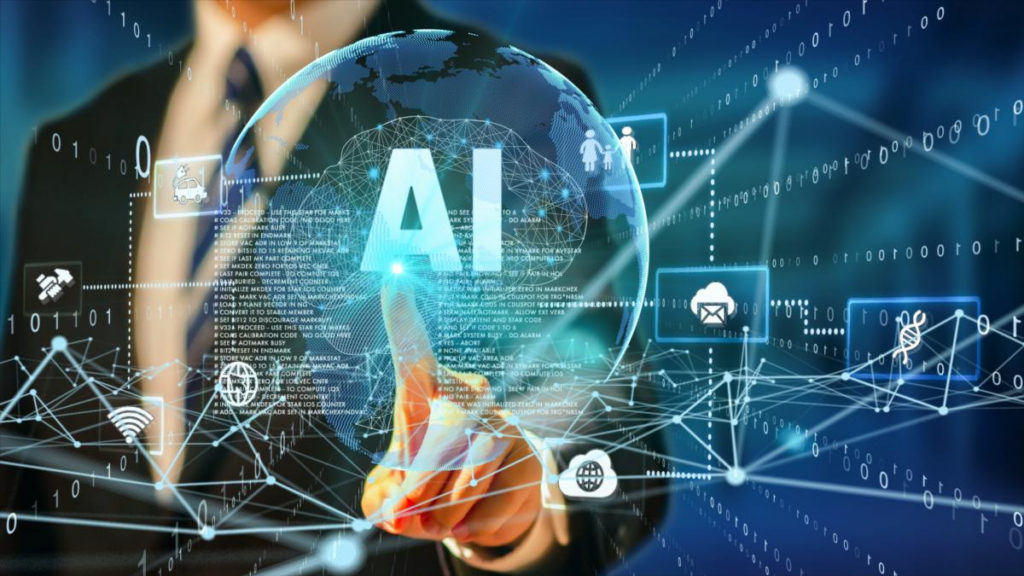
FIAM Montreal AI and its partners organized a conference where top investors, domain experts, and professional associations came together[1] to discuss the successes and frictions of applying AI in Capital markets.
Three broad themes emerged from the keynotes by Nicolaus Henke, Manoj Saxena, Yoshua Bengio, Bryan Kelly, and the discussions moderated by Bill Kelly, Apurv Jain, and Anne-Sophie van Royen– Build, Adapt, and Certify.
- Building a tech platform for enabling data handling and AI is now table stakes for asset management.
- Adapting the organizational structure to capture the thousand small distributed improvements that AI brings is necessary to use the platform effectively.
- Continuously certifying the processes against bias and the current lack of robustness is necessary to build the trust within and outside the organization.
Below we discuss these themes in greater detail.
#1 BUILD. AI/ML Platform is Now Table Stakes for Asset Management.
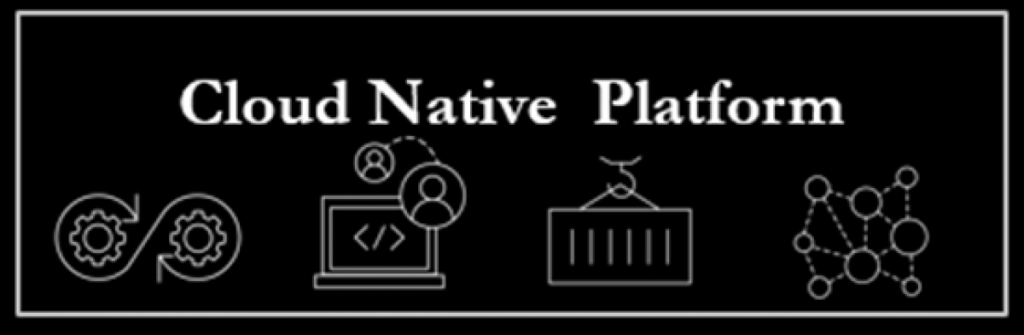
As Nicolaus Henke, the former chairman of Quantum Black and Senior Mckinsey Partner Emeritus, pointed out in his keynote that while the potential of AI is tremendous (according to him it can increase the GDP by $10 to $15T if fully utilized) with several incredible applications like Alpha Go, Protein Folding, and virtualizing testing in sports, it has been quite difficult for organizations to fully adopt – only 28% of the companies have successfully adopted and scaled AI and most have stalled.
What makes adopting AI hard? 1000 small distributed improvements and dealing with lots of data. There are two structural reasons for the stalling in AI scaling – the first is that the opportunity is incredibly distributed with many many use cases – a thousand small alphas if you will, rather than one giant alpha that one can assign to a team, and the second is that the model is only 5% of the story and handling messy data is 95% of the story.
Platform. From a technical point of view, both the data cleaning and modeling capabilities necessitate an ability to rapidly scale data pipelines and as well as apply an ML/AI tool kit quickly. The technical implication of a thousand small alphas distributed across the organization is also a common set of tools, protocols, and vocabulary to be able to capture and process these. When one puts together a common set of fast, super-scalable data and ML tools along with protocols to generate trust that should be used by a varied group (the ones who will capture the thousand small alphas) – one inevitably ends up with a platform. In essence any platform is a scalable piece of infrastructure with specific protocols that makes various users more capable, speeds up the organizational workflow and makes the overall pie of possibilities bigger. Without this type of tool-kit one is effectively “taking a short Big Data and ML position”
Industry leaders’ success and frictions in real life. From the asset management perspective, industry leaders like AQR, Man Group, Two Sigma, Blackrock, and Fidelity shared what successful and varied endeavors their internal tech and ML platforms made possible – from modeling order flow and short-term predictions (0.5 to 1 hour) where the data are very rich, to complex ESG and climate change modeling, to modeling private markets that lack information, to NLP on financial documents.
Examples of challenges faced are faster signal decay of 300 signals rather than the 3 older “factor model” type signals, and the significant effort required in obtaining and dealing with massive data. These technical challenges are on top of the perennial challenge – the profoundly dynamic nature of markets; the non-stationary and reflexive nature can make investing an almost an adversarial game where typically if ‘everyone’ thinks it is a good idea, it may cease to be one. This inbuilt market dynamism is unlike other fields of AI applications where in image recognition a cat stays a cat and not does morph into a dog even with a billion pictures or applications.
#2 ADAPT. The Human Aspect – Org Transformation.
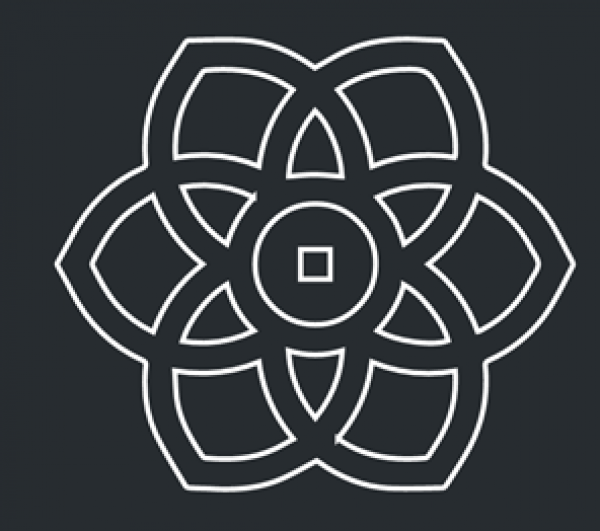
Capturing these 1000 small improvements require organizations to morph. The workflow required is now more complex and necessitates data engineers and scientists to play a major role alongside domain experts and stakeholders. Not only does this require recruiting this data talent but also creating new jobs and career paths to keep them.
Protocols. Within the expanded organization – new protocols of interaction that can build trust and reduce potential organizational entropy are essential – for instance tracking and versioning data and code, that make it easy to monitor data and model quality via dashboards are necessary. Dashboards connected to live data pipelines where provenance can be easily ascertained and experts can be a part of the “cleaning” process will be more useful than a thousand cuts of ‘random data’ put together on an ad-hoc basis.
Mitigating replication errors? There are two main types of replication errors – the first are the simple “computation” types like the Reinhart Rogoff excel embarrassment, and the second are when the promised or in-sample performance of purported factors cannot match the worse out of sample performance due to practices like p-hacking. Having a code-based and audited data pipeline that makes it easy to verify all the analysis steps can at least reduce the computation errors, and hopefully mitigate the second one by providing more visibility into the research process followed.
Org Alignment. More than protocols and data specialists, the key to success is top management aligning around the vision and picking specific focused areas for initial success. For instance, in America’s Cup – a competitive sailing event – there are almost an infinite number of potential variables to focus on. Yet, Team New Zealand and Mckinsey’s Quantum Black chose to focus specifically on using AI methods (such as reinforcement learning) to speed up and improving the hydrofoil design for their boat via a sailing simulator, that played a role in their 2021 triumph.
“Human alpha” or man-machine collaborative efforts to speed up domain experts are quite popular at the top asset managers. Some sample applications include – reducing the time to screen stocks by 50%, two week turn-around time to answer complex deal making questions in private markets and real estate, as well as increasing the potential deals or the improving the top of the funnel. Once again, the crucial practices that make such efforts work are engineers, scientists, and domain experts “sitting together”, code reviews to enable trust, and working along the lines of human expert decision makers.
Canadian Pensions. Some of Canada’s top pension funds shared the various internal initiatives being implemented to bridge the gap. Cross-functional teams with top business sponsors are becoming more common, and many different and sophisticated applications ranging from regime switching, to risk management to smart searching and enabling discretionary managers to generate better ideas are being tried.
#3 CERTIFY. AI Should Earn Trust Not Just Improve Access to be Truly Democratic.
Vast Democratization Potential. AI, a proliferation of data and cheap computing have enabled provision of tools and services to far wider group of people. For instance, as Jane Buchan, CEO of Martlet Asset Mgmt., remarked – the super wealthy always received personalized service – their wealth managers would even know the names of the clients’ dogs! But with technology, now roboadvisors can help a wider group of clients invest and even personalize the service by anticipating their moves in turbulent markets and advising them appropriately.
Trust. Manoj Saxena, the Chairman of Cognitive Scale and Responsible AI Institute, pointed out that “Trust is the foundation of the digital economy.” For instance, it is great to have faster loan approval but when LGBTQ people or minorities get denied loans more often (everything else equal), it showcases the biases and unfairness of our new tools. This means AI is a strategic and social issues with substantial context dependence – one where models and data are not separate. Most organizations are not set up for the line of defense model that is needed to accomplish lower bias. Manoj also shared a responsible AI framework that many organizations endorse and several transformative initiatives his “Do-Tank” responsible AI is undertaking.
A Young Technology. Deep learning legend and Turing award winner Yoshua Bengio pointed out that AI is a “very young technology that has only moved out of university labs recently.” It is hard for governance to come up with precise rules for a fast-moving technology. An insight Yoshua shared is that governance needs to mimic human intuition and classic AI fails because humans cannot fully explain what they are doing. The weakness of current AI is a “lack of robustness” – for instance when the same model is applied to another country or type of data, the results fall off.
Our responsibility. All new technologies bring immense possibilities and as a society we have to decide whether they go in the colonial direction (data colonialism) or improve all of our lives. AI with its immense promise is no exception.
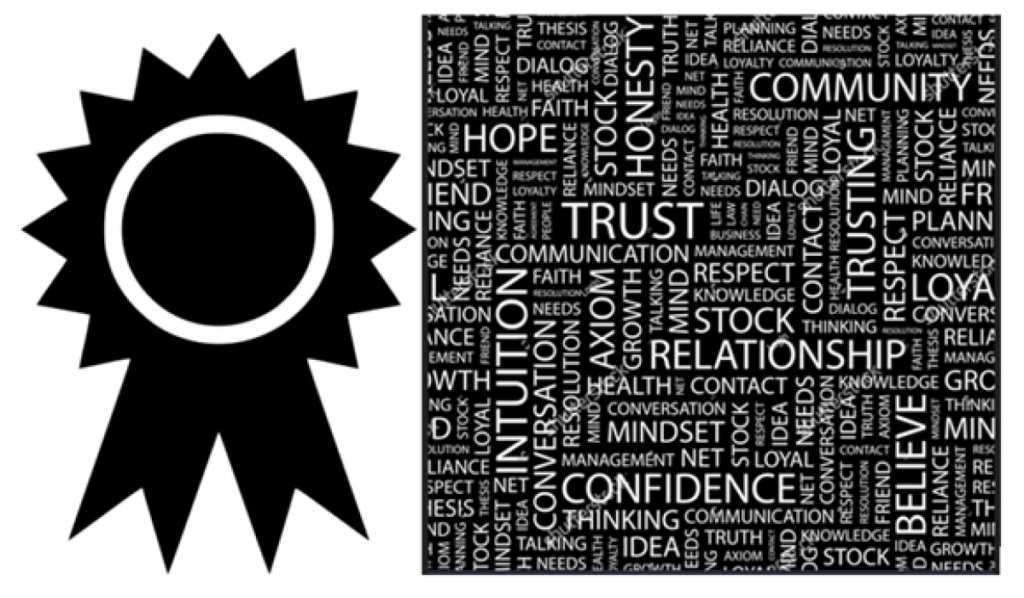
[1] In this conference organized by Claude Perron, the founder of FIAM and Ruslan Goyenko, a professor of finance at McGill University, there were three main categories of discussants:
- Top investors. Industry leaders like AQR, Blackrock, Fidelity Investments, Man Group, and Two Sigma, alongside top Canadian pension investors including CPPIB, CDPQ, OTPP, OPTrust, and PSP.
- Domain Experts. professors from McGill and Yale, alongside experts from Mckinsey and Responsible AI Institute.
- Professional associations. Professional asset management bodies professional bodies such as CAIA, CFA, AIMA, FDP.